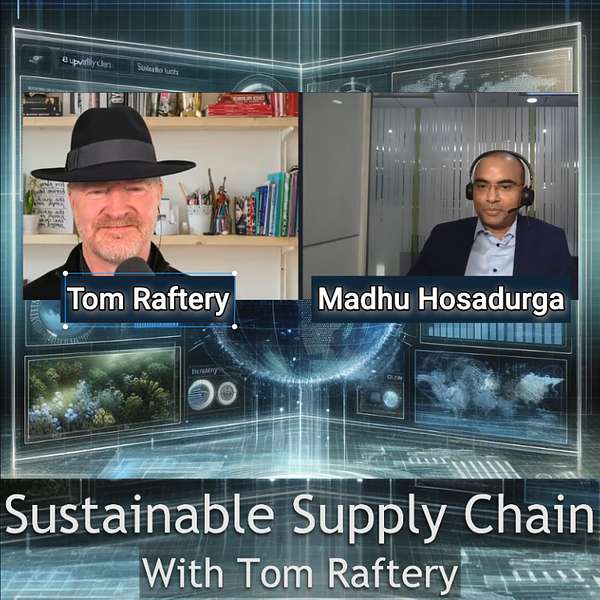
Sustainable Supply Chain
Welcome to the Sustainable Supply Chain podcast, hosted by Tom Raftery, a seasoned expert at the intersection of technology and sustainability. This podcast is an evolution of the Digital Supply Chain podcast, now with a laser-focused mission: exploring and promoting tech-led sustainability solutions in supply chains across the globe.
Every Monday at 7 am CET, join us for insightful and organic conversations that blend professionalism with an informal, enjoyable tone. We don't script our episodes; instead, we delve into spontaneous, meaningful dialogues about significant topics, always with a touch of fun.
Our guests are a diverse mix of influencers in the field - from founders and CxOs of pioneering solution providers to thought leaders and supply chain executives who have successfully implemented sustainability initiatives. Their stories, insights, and experiences are shaping the future of sustainable supply chains.
While the Sustainable Supply Chain podcast addresses critical and complex issues, we aim to keep the discussions accessible, engaging, and, most importantly, actionable. It's a podcast that caters to a global audience, reflecting the universal importance of sustainability in today’s interconnected world.
We are always eager to hear from our listeners. Your feedback and suggestions are invaluable to us, helping shape the podcast into a platform that truly resonates with its audience. Feel free to reach out via email or connect with us on social media to share your thoughts, ideas, or just to say hello.
Subscribe to the Sustainable Supply Chain podcast and be a part of this crucial conversation. Together, let's explore how technology and innovation can lead the charge in creating more sustainable, responsible, and efficient supply chains for a better tomorrow.
Sustainable Supply Chain
Unlocking Efficiency: How AI Transforms Global Supply Chains
Hello, and welcome back to another insightful episode of the Sustainable Supply Chain Podcast. Today, I had the pleasure of speaking with Madhu Hosadurga, Head of AI Internal Offers at Schneider Electric, who shared his extensive expertise on integrating AI into supply chain management to drive sustainability and efficiency.
Madhu detailed the multifaceted approach Schneider Electric employs, focusing on ecological, economic, resilience, and social dimensions of sustainability. He highlighted how AI has revolutionised their operations, from improving energy consumption forecasting to optimising inventory and enhancing quality control across their global factories.
A significant point of discussion was the role of AI in making Schneider's supply chain not only more efficient but also more adaptable to unforeseen challenges. Madhu shared how AI-driven strategies have led to better planning and a remarkable ability to maintain high standards even amidst global supply uncertainties.
We also touched on the future of AI in this sector, including the integration of large language models which can manage and interpret extensive data sets, further enhancing decision-making processes.
If you're looking to understand the practical applications of AI in enhancing supply chain sustainability, listen in!
For further insights and discussions, connect with Madhu on
Elevate your brand with the ‘Sustainable Supply Chain’ podcast, the voice of supply chain sustainability.
Last year, this podcast's episodes were downloaded over 113,000 times by senior supply chain executives around the world.
Become a sponsor. Lead the conversation.
Contact me for sponsorship opportunities and turn downloads into dialogues.
Act today. Influence the future.
Discover how technology is reshaping our lives and livelihoods.
Listen on: Apple Podcasts Spotify
Podcast supporters
I'd like to sincerely thank this podcast's generous supporters:
- Alicia Farag
- Kieran Ognev
And remember you too can Support the Podcast - it is really easy and hugely important as it will enable me to continue to create more excellent episodes like this one.
Podcast Sponsorship Opportunities:
If you/your organisation is interested in sponsoring this podcast - I have several options available. Let's talk!
Finally
If you have any comments/suggestions or questions for the podcast - feel free to just send me a direct message on LinkedIn, or send me a text message using this link.
If you liked this show, please don't forget to rate and/or review it. It makes a big difference to help new people discover it.
Thanks for listening.
Do not underestimate the complexity of change management and the process chain that would be needed when you embark on an AI transformation. So, while AI can definitely help, The success and failure depends on your ability to manage the change from people and process perspective
Tom Raftery:Good morning, good afternoon, or good evening, wherever you are in the world. This is the Sustainable Supply Chain Podcast, the number one podcast focusing on sustainability and supply chains, and I'm your host, Tom Raftery.
Madhu Hosadurga:Hi everyone, and welcome to episode 13 of the sustainable supply chain podcast. My name is Tom Raftery, and I'm excited to be here with you today sharing the latest insights and trends in supply chain sustainability. Today, we're talking to Schneider Electric and we're hearing about how they made their own supply chain more sustainable. And in the coming weeks, we'll be talking to. Trade Beyond around regulations and ESG, we'll be talking to ProducePay around food waste and how to minimize that. And we'd be talking to AIola a company working in the voice, natural language processing space. But back to today and without further ado, I'd like to introduce my special guest today Madhu. Madhu, welcome to the podcast. Who would you like to introduce yourself? Hey Tom thanks for inviting me here today. Very good morning to you. A quick introduction of myself Madhu Hosadurga. I've been in the statistics, BI, big data, AI for close to 22 years now. Started my career with General Electric as a Six Sigma specialist and then worked at a company called Wipro Technologies in again, you know, the big data space helping many Fortune 500 companies in US, UK, Europe. And then I wanted to do my MBA, went to Oxford. Pursued my MBA and then after that started my startup called Oxalytics back in 2014 when AI was still like not very popular subject. And after that ran the startup for about four years and then I was consulting to Schneider as a customer and that's how I ended up joining Schneider in 2018. So it's been five years since Schneider and right now, I head what we call as AI Internal Offers which is primarily helping our functions, operations within the company, leverage AI for efficiency, employee experience, as well as customer satisfaction. That's my current role currently based in Bangalore, India.
Tom Raftery:Nice, nice. And just Madhu, for people who might not be aware, can you give us a few words about Schneider? I know Schneider Electric for a long time. I was in the North American headquarters in Andover back in 2014 for the official opening, but not everybody knows who Schneider Electric are. So give us a few words on Schneider, please.
Madhu Hosadurga:Schneider is a 150 year old company. Headquartered in France, but I need to tell you, it's not Schindler, Schindler is another company and we don't make elevators. So we are a company who are leaders in the energy and industry automation sector with close to about 150, 000 employees across the globe and operations in about 50 different countries. And yeah, you know, we are in the right space, I believe making a big impact in the sustainability space.
Tom Raftery:Nice. Yep. Yep. Absolutely. So you lead the internal offers around AI for Schneider. Tell me a little bit about that, and bearing in mind that this is the sustainable supply chain podcast. So maybe if we can keep the conversation around supply chain and sustainability, what is Schneider doing around AI, sustainability, and supply chain?
Madhu Hosadurga:Yeah, thanks for that. Tom, supply chain is a subject which is very close to my heart, especially in Schneider because when I joined Schneider, I was heading AI for supply chain, and then after setting up that competency, I moved on to the current role, which is a more corporate role across all the functions. Definitely, you know, I, I can throw some light on how AI helped Schneider, especially in making our supply chain sustainable. And in fact, I need to tell you, we are rated as one of the best supply chain in the world. This is probably consecutively third year we got this from Gartner, right? And also I need to tell you, Schneider has one of the most complex supply chain in the world. The reason is we operate close to 200 plus factories across the world. And then these are like really large smart factories. And our supply chain alone has 80, 000 plus people. And we ship about 150,000 orders every single day. And you won't believe we offer 400,000 products to our customers. So, yeah, it's a very complex supply chain yet we are the best supply chain rated by Gartner proudly for the third year in a row.
Tom Raftery:Okay. And how does AI help with that?
Madhu Hosadurga:See before I jump into how AI is helping making our supply chain sustainable, I would like to probably touch upon how I define sustainability, because when I give you examples, you may be able to appreciate them better, right? Sure. So for me, sustainability in supply chain, I can categorize them into three dimensions. One is ecological sustainability, second, economical sustainability, third one, being resilient, and fourth one being social. So these are the four dimensions when I talk about sustainability in a supply chain. Okay. Okay. Because usually people, when, when we talk about sustainability, they are only referring to the ecological effects, the global warming and stuff like that. But here, we really mean being sustainable as simple as that. Whatever we do, can we sustain it forever? Right, so that's the sustainability for us. So now if I have to give you some examples, let's go one by one. So if I talk about ecological sustainability, so what is ecological sustainability? Basically being very eco friendly, right? So that's what it means, right? Making sure we consume less and being very responsible when it comes to our, planet. Yeah. Where can AI help? Again, even before where can AI help, let's talk about trends in the ecological sustainability. For example, one is when we talk about consume less energy is a major part of you know, being ecological, right? So you're moving to renewable energy is probably one of the best move for sustainability. Second circular economy where you can reuse and consume less end of the day. Yeah. See, in both of these aspects, AI can help. I'll give you specific examples. Now, when it comes to energy, we use AI for forecasting our energy demands. Right, for next month, next quarter, six months, next year, so that way we are better positioned to plan our needs and how we saw them, like, you know, whether it's renewable or alternate source of energy. Second, in terms of circular economy, AI can again help in better planning, right, through its ability to forecast the demand through its ability to increase the yield of what we produce, reduce the wastage. So it can really help us consume less yet deliver more. Right. So this is how I see AI is helping us in the ecological impact. Similarly, if I move to the next dimension, which is economical, right, so what is economical? End of the day, keeping cost under control. That's what we really mean by economic impact, right, or economic sustainability. See, AI can help keep cost under control in many ways. Coming back to, again, supply chain, it can help us automate many processes, right? Which definitely helps us keep the cost under control, being consistent, being you know, automated. Second, it can really help us plan our inventory better because in any supply chain, the biggest cost is holding the inventory, producing and holding the inventory, right? Again, not only within a company, I'll tell you, you know, this inventory problem is much bigger than what we think. See globally, at least, you know, from the reports I have read close to 10 to 20 percent is a dead stock, which is very significant considering how much we produce globally. So imagine A good percentage of that is just obsolete. So AI can really help forecast these demands better and then help us optimize the inventory we hold at any point in time. And then of course, AI can help us improve the quality, improve the yield, better workforce planning. And also, in fact, another point which I forgot to mention in the ecological dimension is packaging. AI can really help us optimize how we package. Today, I'll tell you the biggest threat for our planet is so much packaging material used because of, you know, online shopping and kind of ease of shopping anywhere, everywhere. Right. So now AI can actually even help optimize your packing and we use it. In fact Schneider has a certain number of standard packaging sizes, and that's actually optimized using AI. How many size we need, where, which DC is all recommended by AI. Otherwise, it's not easy humanly to decide those, you know, configurations because you need to consider you know, terabytes of data before you come to those recommendations. Yeah. And also we use AI to simulate what kind of packaging is needed, how much can go into a truck stuff like that. So I think it's it's interesting way to use to reduce our packaging or optimizes our packaging and then in turn contribute to a better planet. So now coming back. I covered economical impact and how AI can help. And the third one we talked about is how can we make our supply chain resilient? Right? Because if you want to sustain supply chain, you have to be resilient, right? And you know, the world has become a very unpredictable space in the sense, as more and more supply chain is becoming global, you have a part produced in India, another one produced in maybe, you know, another part of the world and they are assembled somewhere else and sold to somewhere else. Right. Imagine the complexity of the supply chain. And then on top of that, you, you always are faced with geopolitical issues. You are faced with you know, financial, economical issues. You're faced with shortages of resources. So supply chain is constantly faced with a lot of uncertainties and more so in the last three, four years, and it's ever increasing. So now for us to sustain our operations, we have to be very resilient and this is where, again, Schneider uses AI to become more resilient. For example, we use AI to decide how much safety stock to hold in which location. Again, considering all the variability in the market and the issues which are not foreseen. We also use AI to better planning again, as I said, again, planning is an area which touches all of those dimensions. And also helps us make our supply chain resilient. And then the last one, last dimension which we talked about was socially sustainable, meaning we need to ensure safety of our employees, safety of our partners. Again, we use AI to ensure safety in many applications. We use actually computer vision to monitor any hazards. Even during COVID, we were using computer vision to make sure everybody is wearing their mask and stuff like that. Right. And also we use AI and automation to ensure there is a good work life balance for our people, which is also very important for us to sustain our operations. Otherwise, burnout is not good to sustain any operations. In fact, I'll tell you again you may have heard, in finance sector, banking sector, month end, you have quarter end, you have year end, they are super busy, right? Very common to hear, but then nobody tells you supply chain people have day end every day, meaning every day is a day end, meaning so much planning need to be done for the next day. So the amount of pressure the supply chain people go through with all these uncertainties is humongous. And this is where we are trying to leverage AI to make the lives of our people more predictable, right? And, you know, create a more balanced work life. So these are kind of, you know, how we Use AI in these four dimensions to make our supply chain more sustainable. Again, there are like a lot of use cases, which I can tell you you know, maybe let me, let me tell you the examples because some of them could overlap across all these four dimensions. For example, in procurement, we use AI to help our buyers negotiate better price. We use AI to decide what should be the lot size of the raw materials we buy, as simple as that. And then in manufacturing, we use it for predictive maintenance, predictive quality, and also production scheduling, production planning. And then when it comes to logistics, we use it in the route optimization, DC layout plan, forecasting our shipment needs. And we in fact try and avoid air shipments just again, mainly from an ecological reasons. So that's where we forecast the needs and plan better for more ocean shipping than the air shipping. And then in the customer satisfaction area, we use a lot of NLP techniques to hear our customer voice. And kind of help us to help them to service better. Again, the list goes on in fact, maybe if I have to kind of throw a number, maybe we are working on 20, 25 highly impactful AI use cases within supply chain alone. Of course, the same similar number follows in finance and e commerce and sales, marketing, all those areas. But since today's topic is more supply chain, I'm saying it's around 20 - 25, highly impactful use cases, which are fully live you know, being used by our operations on a daily basis. Hope that answers your question on use of AI within supply chain and sustainable supply chain.
Tom Raftery:Sure. Yeah, no, absolutely. And just to clarify for people listening, these are not off the shelf solutions that you've brought in from some AI vendor. These are homemade, right?
Madhu Hosadurga:Yeah, in fact, it's a very good point you bring up, Tom. First of all, to answer your question, yes, all homemade. I'll tell you why. See, Schneider Electric as an organization is is an organization which has grown through a lot of acquisitions, right? And and our IT complexity. is probably much bigger than the supply chain complexity I mentioned to you, right? So, because we kind of have close to, you know, 30, 40 ERPs, which we run at any point in time, again, thanks to the acquisitions and mergers and, you know, operations in different countries. So, any off the shelf product may not work smoothly for us. So, and secondly, we also believe this is an area which is yet to mature. So there are not also many off the shelf products available in the market today which can help our complexity and our ambitions. So mostly they are developed in house. Of course we leverage some off the shelf platforms like, you know, SageMaker, Databricks and stuff like that. But then we build on top of it. Yep, that's the reality. We have to build them in house from the scratch.
Tom Raftery:Okay, and For people who are listening, who are wondering how they might embark on something similar themselves, can you, I guess, speak just from Schneider's perspective on what were the, what were the top delivering solutions in terms of, you know, which ones gave the best bang for the buck?
Madhu Hosadurga:Again coming again from a pure supply chain point of view, I think the most popular use cases and the ones which we use and they make a significant impact or, of course, demand forecast because supply chain is nothing but, you know, it's a equation of demand and supply at any point in time. Inventory optimization is another very good use case which I think every company should use mainly because it has a huge impact on sustainable planet. Third, energy consumption. So they need to use AI to optimize the energy. Again kind of simulate forecast, renewable versus non renewable and kind of take that to plan the production and stuff like that. And then there are other use cases, but the impact depends on the nature of business they are in, right? For example, packaging optimization, route optimization, all of this depend on how complex their supply chain is. But inventory is definitely is a top one. Forecast, demand forecast, which I'm pretty sure many companies already use it is, is another one. Yep, those are probably the top ones which people can use. And then in manufacturing, of course, predictive maintenance, AI driven quality, AI driven yield. In fact, Schneider even acquired a company called Aviva, which is a leader in the digital twin space that really helps digitize manufacturing and also bring the data from connected devices to be used for AI purposes, right? So manufacturing can also use ai to get better in terms of yield and quality. Again, as I say, in each of the supply chain topics, we can have multiple use cases, depending on the nature of business you are in.
Tom Raftery:Okay. And how, how in manufacturing are you increasing yield and quality by using AI? How does that work?
Madhu Hosadurga:So, for example if I have to give you an analogy, see many of us drive car, right? So the OEM, the, the equipment manufacturer or the car manufacturer usually say you drive between X mile and Y mile to get the best fuel economy or efficiency, right? While that's true in an ideal condition, but come to a city like Bangalore, the traffic is different, the temperature is different, the AC is running at a different temperature. So the local context may not respect the guidelines given by OEMs. And this is where something like AI, which can take into consideration how the car is behaving historically, and then can make recommendations to the user saying, Hey, instead of 40 to 60 miles per hour, if you drive, let's say 20 to 30 miles per hour, with the AC at 22 temperature, you may get your best efficiency for your fuel, right? And this is how AI can help. It can make recommendations based on the local parameters or local context. So this is one analogy of how you can make most out of your fuel. Similarly, in manufacturing context every production line has a lot of controllable parameters, like you can set your pressure, you can set your temperature, you can set your you know, torque, so on and so forth. Like any production line at any point in time may have you know, 15, 16 different parameters and imagine most of these parameters are set based on the OEM recommendations throughout the world, right? And some of it may not be applicable given the local context and humanly, it's not possible for someone to trial and experiment different combinations on a given point in time and keep changing them. It won't work. So this is where. They can actually have an AI, now it could even be real time. In fact, when we started, it was not necessarily real time. We would run it once a week, once a month, and then recommend the ideal parameter settings. But then now it can literally be real time and also self-healing. You know, you can actually use AI to monitor these parameters, control them, self-heal as the situations change, yet give you the best yield and the quality, right? So, this is where I think we were is helping our customers. This is where ai is gonna help customers in better quality and yield. Again, leads to a good, sustainable manufacturing. Sure,
Tom Raftery:sure, sure. Because I'll tell
Madhu Hosadurga:you at any point in time just to add to that, the wastage is not small across the world. When we talk about manufacturing, we still have 5 to 10 percent wastage you know, yield no more than 85, 90%. So there is still room to improve them. And this is where I think AI can help a lot.
Tom Raftery:Yeah. I was going to ask about that, about quality control and waste. How is AI helping there and how can it help more?
Madhu Hosadurga:Yeah. Again, I think you know, the, the self healing parameters what I talked about is a great example of how AI can help reduce the quality issues, right? See, quality issues usually happens because of wrong inputs, right? See, for example, in Schneider, I'll tell you, we have one use case, which it's an AI use case, which monitors the thickness of the welding, right? Okay. And, and if the thickness of the welding goes wrong, you end up in a bad product, right? And that thickness depends on many parameters, right? Imagine, humanly, if someone has to monitor each of them every minute, every hour. It's not practical, not scalable, not sustainable. So here we have an AI which keeps monitoring, make sure it's within the, you know, the limits what we want it to be, right? In fact, we kind of embed you know, the AI logic into the PLCs, which call it which, which kind of controls these parameters and make sure the quality are within the limits.
Tom Raftery:Fascinating. Fascinating. And where to next for AI and supply chain? What do you see as the kind of the big next steps forward? Because we've seen, I mean, larger language models have really come to the fore in the last 18 months. I'm not sure how big an impact they have on the likes of manufacturing, but what do you see as we move forward the next three, four, five, 10 years, where do you see the big impact happening for AI and supply chain?
Madhu Hosadurga:In fact, I'll probably answer it in two parts. One is AI, the traditional AI itself is yet to be used by many manufacturers yet. Right. It is still, I still see many companies are still at more PoC level, like proof of concepts. They have not been able to scale and stuff like that. I think the next trend I see from traditional AI perspective is now people are starting to look AI at scale. And they start to look AI part of their operations, no more as an innovation, no more as just a proof of concept, right? So that's one trend where people start to leverage AI. Now, of course, the recent trend is more on the large language models. Right. So again NLPs have been there for many years, natural language processing, of course, LLMs have taken it to the next level for sure. So there are many use cases in the supply chain space that can leverage LLMs. I'll tell you how Schneider is using LLMs in the context of supply chain. For example, in procurement, we deal with thousands of suppliers and their contracts. So now we use LLMs to manage those contract documents. End of the day contract is you know, a fully language oriented document. So we use LLM there. Similarly, we are using LLMs to use our debug reports. Like every time something goes wrong in our manufacturing, across the globe, across 250 factories, we have a lot of machine logs. And engineers giving a lot of their analysis in, you know, pure language. So now we use LLMs to summarize, see if there is any kind of synergies any kind of overlaps, commonalities and things like that. Similarly, the whole knowledge space, whether it's you know, knowledge of previous resolutions, issues common across the globe. So LLMs, we kind of see in two different dimensions. One is more of managing the knowledge and another one, more of assistants. Like assistant is, Hey, can you draft me a contract for a certain type of supplier, or, Hey, can you help me draft an RFP for procuring this particular parts? So one as an assistant, another one more as managing knowledge that knowledge could be debug issues, manufacturing defects, resolutions, customer complaints, so on and so forth. So I definitely see a lot of good applications in the supply chain space when it comes to LLM. Even, you know, scraping the web to analyze geopolitical trends you know, shortage issues, the semiconductors, shipping issues. There is so much happening on any given day in the supply chain world, so LLM can really help there. Nice. So these are the two kind of trends I would say. One is more AI at scale, in real, in parallel to the applications, ERPs, second one, leverage of LLMs for knowledge and assistance.
Tom Raftery:Okay, great. We are coming towards the end of the podcast now, Madhu. Are there, is there any question I didn't ask that you wish I did or any aspect of this we haven't covered that you think it's important for people to be aware of?
Madhu Hosadurga:Again I think we've kind of covered most of how AI is used in the supply chain or can be used in the supply chain to make it more sustainable. But again, there are a few takeaways which I would like to highlight based on our own learning. We've been trying to leverage AI for close to now five years in Schneider Electric, of course, there was AI before that as well, but this five year is more of very organized, well invested you know, structure. So, definitely, I think, you know, top takeaway to the listeners is now, when they talk about AI, they should look at AI at scale. Second, which I think worked very well for us, is not to see AI as just a technical competency. It should be very well integrated with the business domain. In fact, at Schneider Electric, the way we deliver AI solutions is using a model called hub and spoke model, where every use case, we bring people from the technology competency on the business domain, we form a squad, and that squad delivers the use case. The squad has a good mix of business and the technology expertise, right? So this is I think everybody definitely should keep in mind when they embark on an AI journey. And the third one, usually I, I hear people saying to do AI, they don't have the required data maturity. Of course, it's important to have the right data to do AI, but again, that should not become an excuse not to do ai. I don't think there will ever be a perfect data world which one can wait to do ai. It has to go hand in hand. In fact, the way I see ai, AI should drive a better data. Like you you start ai, that's when you kind of start to realize, you know, what is missing, what processes need tweaking. What you know, systems need tweaking and then kind of AI drives some of the transformation, right? And fourth one, do not underestimate the complexity of change management and the process chain that would be needed when you embark on an AI transformation. So, while AI can definitely help, The success and failure depends on your ability to manage the change from people and process perspective. So those are kind of, you know, my learnings in the last four, five years and, and what has worked well for us. I, I am sharing with the audience here.
Tom Raftery:Very good. Madhu, if people would like to know more about yourself or any of the things we discussed in the podcast today, where would you have me direct them?
Madhu Hosadurga:They can reach out to me in LinkedIn. So I'm available with the name Madhu Hosadurga. Happy to take any questions on LinkedIn. Yep, I'm mean, happy to share my experience and learning and be an ambassador of ai, especially in the supply chain space.
Tom Raftery:Fantastic. I'll put that link in the show notes so everyone has access to it. Madhu, that's been fascinating. Thanks a million for coming on the podcast today.
Madhu Hosadurga:Thanks to you, Tom, for inviting me here today. It was a pleasure speaking to you.
Tom Raftery:Okay. Thank you all for tuning into this episode of the Sustainable Supply Chain Podcast with me, Tom Raftery. Each week, thousands of supply chain professionals listen to this show. If you or your organization want to connect with this dedicated audience, consider becoming a sponsor. You can opt for exclusive episode branding where you choose the guests or a personalized 30 second ad roll. It's a unique opportunity to reach industry experts and influencers. For more details, hit me up on Twitter or LinkedIn, or drop me an email to tomraftery at outlook. com. Together, let's shape the future of sustainable supply chains. Thanks. Catch you all next time.