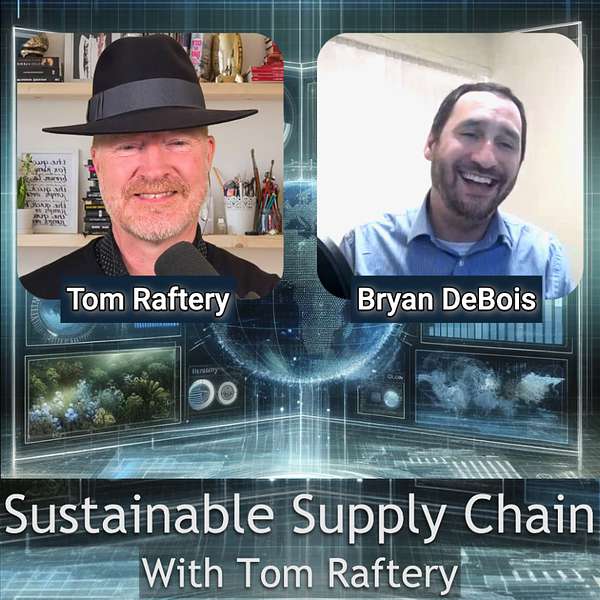
Sustainable Supply Chain
Welcome to the Sustainable Supply Chain podcast, hosted by Tom Raftery, a seasoned expert at the intersection of technology and sustainability. This podcast is an evolution of the Digital Supply Chain podcast, now with a laser-focused mission: exploring and promoting tech-led sustainability solutions in supply chains across the globe.
Every Monday at 7 am CET, join us for insightful and organic conversations that blend professionalism with an informal, enjoyable tone. We don't script our episodes; instead, we delve into spontaneous, meaningful dialogues about significant topics, always with a touch of fun.
Our guests are a diverse mix of influencers in the field - from founders and CxOs of pioneering solution providers to thought leaders and supply chain executives who have successfully implemented sustainability initiatives. Their stories, insights, and experiences are shaping the future of sustainable supply chains.
While the Sustainable Supply Chain podcast addresses critical and complex issues, we aim to keep the discussions accessible, engaging, and, most importantly, actionable. It's a podcast that caters to a global audience, reflecting the universal importance of sustainability in today’s interconnected world.
We are always eager to hear from our listeners. Your feedback and suggestions are invaluable to us, helping shape the podcast into a platform that truly resonates with its audience. Feel free to reach out via email or connect with us on social media to share your thoughts, ideas, or just to say hello.
Subscribe to the Sustainable Supply Chain podcast and be a part of this crucial conversation. Together, let's explore how technology and innovation can lead the charge in creating more sustainable, responsible, and efficient supply chains for a better tomorrow.
Sustainable Supply Chain
Beyond Efficiency: How AI is Crafting a Greener Manufacturing Era
In this week's episode of the Sustainability Supply Chain podcast, I had the pleasure of speaking with Bryan DeBois, Director of Industrial AI at RoviSys, about the transformative power of AI in the industrial and manufacturing sectors. Bryan shared fascinating insights into how RoviSys is leading the charge in applying Autonomous AI and machine teaching to solve operational challenges, significantly reducing waste, and boosting efficiency on the plant floor.
2023 has been a pivotal year for AI, and Bryan's experiences underscore the growing demand for AI solutions that not only enhance productivity but also drive sustainability. He delved into real-world applications of Autonomous AI, from optimising steam usage in baby formula production to revolutionising the glass bottle manufacturing process through vertical integration. These examples highlight the potential of AI to align economic incentives with environmental sustainability, dramatically reducing emissions and resource consumption.
Bryan also touched on the challenges of operationalising these technologies, stressing the importance of embracing innovation and the critical role system integrators play in bridging the gap between AI's promise and its practical implementation.
This episode is a must-listen for anyone interested in the cutting-edge intersection of AI, manufacturing, and sustainability. Bryan's expertise offers valuable perspectives on how embracing AI can lead to a more sustainable and efficient future.
Tune in to explore how AI is not just shaping the future of manufacturing but also paving the way for a more sustainable world. Don't forget to check out the video version of this episode at https://youtu.be/ftB10NEtpiE
For weekly insights and discussions on the nexus of technology and sustainability, be sure
Elevate your brand with the ‘Sustainable Supply Chain’ podcast, the voice of supply chain sustainability.
Last year, this podcast's episodes were downloaded over 113,000 times by senior supply chain executives around the world.
Become a sponsor. Lead the conversation.
Contact me for sponsorship opportunities and turn downloads into dialogues.
Act today. Influence the future.
Podcast supporters
I'd like to sincerely thank this podcast's generous supporters:
- Alicia Farag
- Kieran Ognev
And remember you too can Support the Podcast - it is really easy and hugely important as it will enable me to continue to create more excellent episodes like this one.
Podcast Sponsorship Opportunities:
If you/your organisation is interested in sponsoring this podcast - I have several options available. Let's talk!
Finally
If you have any comments/suggestions or questions for the podcast - feel free to just send me a direct message on LinkedIn, or send me a text message using this link.
If you liked this show, please don't forget to rate and/or review it. It makes a big difference to help new people discover it.
Thanks for listening.
If you think about it, if you have one of these AI solutions, you've got a model. It, it's working great, it's predictive, whatever, until it's on the plant floor and until someone in operations, till a supervisor is trusting it instead of their own experience, their own eyes, ears, you know, years of decades of experience in some cases, until they're using that to make better decisions, you're not seeing any ROI. Everything up to that point was a giant science experiment.
Tom Raftery:Good morning, good afternoon, or good evening, wherever you are in the world. This is the Sustainable Supply Chain Podcast, the number one podcast focusing on sustainability and supply chains, and I'm your host, Tom Raftery. Hi everyone. And welcome to episode three of the sustainable supply chain podcast. My name is Tom Raftery. I'm excited to be with you today, sharing the latest insights and trends in supply chain. Before we kick off today's show, I want to take a moment to express my gratitude to all of our amazing supporters. Your support has been instrumental in keeping this podcast going, and I'm really grateful for each and every one of you. If you're not already a supporter, I'd like to encourage you to consider joining our community of like minded individuals who are passionate about sustainability and supply chain. Supporting this podcast is easy and affordable with options starting as low as just three euros or dollars a month. That's less than the cost of a cup of coffee and your support will make a huge difference in keeping this show going strong. To become a supporter you simply click on the support link in the show notes of this or any episode or visit tinyurl. com slash s s c pod. Without further ado, I'd like to introduce my special guest today, Brian. Brian, welcome to the podcast. Would you like to introduce yourself?
Bryan DeBois:Sure can Tom. So I'm Bryan DeBois. I am our Director of Industrial AI at RoviSys. So we are a system integrator that is headquartered, just south of Cleveland, Ohio in the United States. Although we're a global SI, we've got about 17 locations around the world and about 1400 engineers globally. So we work primarily with industrial and manufacturing customers. In this role as Director of Industrial AI, my, my job really is to bring AI solutions to, those industrial and manufacturing clients. And we, you know, we started this division about five years ago. but I've actually been with RoviSys for about 23 years. And my background, I've actually got a computer science degree.
Tom Raftery:Yeah. Nice, nice, nice. And I gotta think, you know, 2023 was a big year for AI. So, you know, did you suddenly get really busy?
Bryan DeBois:In a lot of ways, yes. Although, you know, over that five year period, we really have seen a lot of steady growth as more and more of these customers start to look for AI to bring those, you know, those, those material gains in. Both in, in scrap reduction and increases in yield and things like that. And AI seems to be the most promising tool, to be able to reach that. Now, of course with 2023, it really was the year of Generative AI, right? And so, I was talking to someone recently last November about ChatGPT, and I was like, you know, it's interesting to think about. We're really only a year into ChatGPT as a phenomenon. It was November of 2022 when it really had its big coming out party, and then they got, you know, a million subscribers in, in a very short period of time and all that. And so, 2023 really was kind of the year of, of Generative AI. And so what's happened then is, is that a lot of customers will call me up and get excited and want to bring me in to talk to them about AI because of what they've seen with the likes of chat, GPT and Dall-E with the image generation and things like that. What I end up telling them though, is kind of pivoting is, is, is that while those generative AI solutions are really good at, so solving knowledge types of problems, the reality it is on the plant floor that we end up seeing a lot more operational types of problems. And there are other solutions, and one in particular that we're, we're pretty heavily invested in is called Autonomous AI, which we can talk about later, but that really is more of a solution to solve operational types of problems on the plant floor.
Tom Raftery:Okay. And I mean, I can see ChatGPT, great for writing, you know, social copy. Great for, as you said, you know, creating nice pretty images for you. I don't see a lot of functionality for that on the plant floor. So what is it that your Autonomous AI can do that the likes of LLMSs and ChatGPT can't?
Bryan DeBois:Yeah. Well, and, and real quick, just, you know, to to talk about maybe some of the, the real limitations that we're concerned about with, with the generative AI on the plant floor is this idea, and, and a lot of people have talked about is this concept of hallucinations. And, and the idea there is, is that LLMs have a really hard time of saying, I don't know. If they don't have the information, they typically will just make something up. Now ChatgPT does a little better job of saying, yeah, that's outside of my, you know, realm of knowledge, but it's been programmed to do that. Most LLMs will just make something up, and the problem on the plant floor. And so everyone's scrambling to try to figure out how to apply this in, in manufacturing. And maintenance is one of the big areas that they've looked at because you typically do have lots of, stores of knowledge and lots of standard operating procedures and lots of history there that you can digitize and then train, you know, an LLM on. The challenge is, is that if you say, Hey, I I've got this machine in front of me and it's having this problem, how do I fix it? Even if that data's not in the LLM, it's happy to say, well, I know exactly how to fix that problem. Take this bolt and, and torque it to this, you know, and you could blow up the plant and, you know, so. That's, that's, that those hallucination hallucinations on the plant floor can, can lead to some really bad outcomes. So I, you know, we're keeping an eye on it. I think that there's still a lot of promise there, but there's still a lot of research that needs to happen. Now if we, if we look at Autonomous AI and what it's capable of, so it's really built on a couple core, technologies. One of them is deep reinforcement learning. So this, this is what came outta DeepMind, which was a Google spinoff. It was around 20 15, 20 16. Um, and it's big coming out was Alpha Go. So this was a, a program built to play Go. And it ended up beating, Lee Sedol, which was a, he was a Go grand master, a human Korean master, and it beat him. And then they went on to build Alpha Zero, which beat our best chess grand masters, and then it beat our best chest software. So if you think about the decades of optimizations that were built into that software, and it beat it handily. So they were really onto something. They built Alpha Star, beat our best StarCraft players. So they're really onto something. And so what, you know, of course. So then over the, years since people have applied that same deep reinforcement learning to different problems, in this case it would be industrial types of problems. And why it's significant is, is that Autonomous AI can do, frankly what our customers think AI can do, which is that it can actually look at the state of a system and say, based on everything I'm seeing, here's your next most optimal thing to do. It's not just gonna make a prediction, which is what like supervised learning does. That's kind of the traditional predictive types of, of machine learning. It will actually make a recommendation. It'll actually say, take this action. And what's interesting is, is if you think about how it's able to beat grand masters and things like that, it has to be able to build long-term strategy so it can make decisions that look suboptimal in the short term because it's working towards a long-term. It's working to win the game. It's working towards a long-term, more optimal solution. And frankly, when I start to talk about that with customers, that's what they get excited about. They go, that's what we need, you know, in this industry.
Tom Raftery:Okay. It's all very well beating grand masters, but how is this helping us with sustainability?
Bryan DeBois:Yeah. Well, and so what's interesting about sustainability is oftentimes it's just a question of aligning incentives. So we've been working with customers, you know, I've been working with customers for over 20 years now on things like optimizing yield and increasing yield, but also on things like decreasing scrap, decreasing energy usage. In our world we talk about, an acronym called WAGES. So that's water, air, gas, electricity, and steam. Those are the primary utilities, the primary inputs besides labor into almost every manufacturing process. And we've been talking about reducing the consumption of that. Now, again, most customers are probably primarily interested in that because of cost reduction. Besides labor, those are typically the most expensive inputs to the process. But if we can align incentives, the, that the gains in, in re reduction of WAGES is the same thing that's going to reduce the amount of, emissions in the future. So, so it's really just about aligning incentives.
Tom Raftery:Okay. And do you have any examples you can talk to where, you know, you've managed to reduce one of the letters of WAGES, or all of the letters of WAGES, and, and, and, you know, have, have reduced emissions as a consequence?
Bryan DeBois:Sure can. So we've got a project that we are doing right now so this is a manufacturer of baby formula. And so part of that process is, is that you, you put, you know, big, trays of cans, big carts of cans into a steamer tort, and it applies steam and pressure to sanitize the cans. Well, the amount of steam that gets consumed by that can be significant. There's also a question of throughput there. So there's, I think they have about eight, retorts that are all working off this same steam generator. And so, What we're looking at doing is, is you, you need to be able to sanitize the cans, but there's a point where diminishing returns where you're, you're applying steam, but the cans have already been sanitized. So again, they're looking at steam reduction and they're looking at throughput increase. But ultimately, from an emission standpoint, from an energy usage standpoint, if we can reduce the amount of steam that's required by by sanitizing them just enough, but not more than is necessary, we can increase that throughput. There's also an orchestration problem there, which is really interesting because, the steam, the system that provides the boiler that provides the steam can't actually run all eight at the same time. So they've got interlocks, which a, which, you know, doesn't allow one steam retort to kick off if another one is, is using it. So that, so there's this whole dance that we can train an autonomous AI brain. So a neural network trained this way is called a brain. We can train a brain to act like this great coordinator to be able to monitor everything that's going on, only sanitize just up to the, the curve, you know, just to kill all the bugs and nothing more, and then be able to increase that throughput, but again, also reduce the amount of emissions.
Tom Raftery:Okay. Nice, nice. And you talked as well about reduction in scrap which seems like a, a different tack towards a, a similar output, similar outcome. Can you talk to me a little bit about that? How does that work and do you have any examples you can talk to on that one?
Bryan DeBois:I do. You know, it's, I don't know, it's a blessing and a curse to, to work in the industry that I do, because I get to go into these, these plants and it's absolutely fascinating. I was, I was someone, there's a show at least in the US called, on the science channel called How It's Made. And it shows, you know the how things are made and I always used to love that show. And so now, you know, in, in my career I get to do that and I get to go into these factories and I get to see how things are made. It's absolutely fascinating. On the downside though, I do get to see kind of the dirty underbelly of manufacturing. One of those is the amount of waste. You know, massive, massive amounts of waste to the point where it's pretty disheartening. You know, if, if you talk, I and I, I, I get the impulse. I talk to friends of mine and they're, they're really worried about recycling and they're really worried about reducing, and I'm like, household waste just pales in comparison to the tons, literal, tons of industrial waste, that we generate every day. So, if we can reduce that, that's a significant win. So, as an example of that, and again, it's about aligning incentives. We worked with a drywall manufacturer. And so the current state of the art is that you, you know, you create these drywall sheets and then, you know, they go and then you do, there's a destructive test. They take a sample from the drywall sheets, they send it to the quality labs, and it takes about an hour. It could take longer if the labs at lunch or whatever might take two hours. So they're still making sheets while those results are coming back from the lab. When they finally get it back, they only then realize, well, we've been making garbage, we've been making scrap, and all of this will go to a landfill. And so then they make the adjustments so that they can, you know, get dialed in and start making good sheets. And this process, you know, repeats. Well, we were able to build a computer vision system because it turns out that as the drywall's drying, there's some certain visual indications that we can detect, with certain cameras that we can actually, predict what the final quality of those sheets is going to be. Before the hour long wait for quality, and we're talking, you know, effectively near real time in minutes, we can, tell the operators that they're, you know, this, the quality is, is starting to trend downward and they can make the adjustments in real time to get back to making good product. Now again, that drywall manufacturer did it because they wanna make more product in, increase their throughput, but the, the side effect of that, if we align the incentives correctly, is, is now we've instantly reduced the amount of scrap that this company generates. And the, you know, the ROI that this company has told us that they're seeing is, is massive, hundreds and hundreds of thousands of dollars. And then of course, tons and tons of scrap, drywall that they're not making anymore.
Tom Raftery:Nice. Nice, nice. You mentioned as well on the intro call vertical integration and ways that that can help. Talk to me a little bit about that and give me a couple of examples if you can.
Bryan DeBois:you Yeah. So we've got a customer right now. They build, furnaces and the process for, for creating glass bottles. And so the process for creating glass bottles, the current state of the art is you have to have a furnace that's about the size of a two story building. It's a very involved process. Obviously a ton of energy is required to do this. Obviously a giant, footprint in terms of the actual building, and lots of staff. They are developing a process right now that will use. It. It's a whole new way of, of, of building, glass bottles. And it's so small that it can, it's a effectively the size of, of a couple trailers. And they, the idea would be that instead of having to make these bottles in one plant and then ship them across the country to be filled somewhere else, this new process is so small that they can basically have it on site. And so a bottler could basically have sand coming in, make bottles, and then fill them right on site. So you talk about all of the upstream downstream transportation that's required there. All the energy that's typically consumed, all the, the hours and everything, you know, and then, you know, typically like the cleaning process of, of these bottles and everything, that would not have to happen if you knew that they were pristine, they were coming right off of the line and you could fill them right away. So that's that vertical integration. I think everyone's moving towards that. We've seen more and more metals, companies that are moving towards vertically integrated steel mills and, and aluminum mills and things like that. In the case of that bottle manufacturer, they're actually using autonomous AI then to, so this thing would ship, they would actually sell this to you know, bottlers and things like that. And, and the autonomous AI that, that we've designed would actually run that system. One of the challenges when you're building something that's never been built before is how do you get operators who actually know how to use it? So, in the case of that process, they only have two operators in the world because it's brand new, who are experts at being able to make these bottles? Well, so what we're doing is, is we're extracting the information from their heads, we're using a process that's also part of that Autonomous AI, umbrella called machine teaching. That's where we actually take all that expertise from those SMEs, and we actually build kind of like a workflow where you sit where it says things like, it makes decisions on, okay, you know, if the line's running hot, you turn this knob. And if the line's line's running cool, and this is all expertise that we got out of their heads, but we build it into this graph. And so then when we let the DRL loose on that to learn. It actually, you know, it can leverage those heuristics, those best practices from the best operators. So for free, you also get this, this thing that a lot of our customers are asking us for is how can we capture the expertise from our subject matter experts before they retire, before they walk out the door? This technique really is able to do that as well,
Tom Raftery:So basically you're cloning people,
Bryan DeBois:in the best way possible.
Tom Raftery:Fantastic. Fantastic. Any other, success stories you can talk to about your clients and big wins you've had in sustainability, or emissions reductions?
Bryan DeBois:Yeah, so, One of the areas that we're applying this technology to as well is we've got a natural gas supplier. They're primarily out of Canada, but they also operate some of the United States. And so we built an AI that can actually predict the amount of demand for their natural gas. So the idea is, is that it's expensive. It, you know, it takes energy and anytime you expend energy, you're increasing emissions. So it takes a lot of energy to move this natural gas. Especially if there's unexpected demand. And so now you are, you're taking herculean efforts to try to get that natural gas to where it needs to be. If instead you can predict a week ahead of time that that natural gas needs to be at certain parts of the country, as you would expect, things like, you know cold fronts and as well as historic demand. And it takes all of that into account and upcoming weather. It takes all that, that AI takes all of that into account and then says, okay, you need to move this much natural gas here to here. So if we can, again, if we can get ahead of that, if we can move that when energy is cheaper, when emissions would be less, we can get ahead of that. And, again, I think that that's, that, that's that ability AI has to both, decrease costs for, for our clients, but then also to decrease things, like energy emissions.
Tom Raftery:Nice. Nice. And what would you say have been some of the biggest challenges you've encountered in operationalizing technologies for sustainability? And, you know, how would you, how have you overcome them?
Bryan DeBois:Yeah, well, from an operationalizing standpoint, we're, to toot our own horn because we've got 30 plus, we've been around since 1989. So because we have 30 plus years experience working in the plant floor, we actually are able to overcome a lot of the objections and a lot of the issues that maybe some of the bigger consultancies or the bigger vendors run into. We're very good at organizational change management, especially with those people on the plant floor. You know, if you think about it, if you have one of these AI solutions, you've got a model. It, it's working great, it's predictive, whatever, until it's on the plant floor and until someone in operations, till a supervisor is trusting it instead of their own experience, their own eyes, ears, you know, years of decades of experience in some cases, until they're using that to make better decisions, you're not seeing any ROI. Everything up to that point was a giant science experiment. So, we've been able to leverage that. And really, so the operationalizing piece of it is, is, is something that I think we, that we do really well at. The challenges really come from the fact that this is just an manufacturing is just a risk averse industry and for good reason. And so, they tend to be five to seven years behind the rest of the world in terms of adoption of technology. So you run up against that issue. And then you just have, companies that are just not that forward thinking. They've been doing things a certain way for a long time. I was reading an article and I won't mention them by name, but there's been a recent giant acquisition of a US Steel, manufacturer recently, which has made a lot of headlines and I read it an analysis of it. And, and you know, the big thing that they said in the article was that, they, this particular company was the last to adopt a lot of the new technologies. You know, looking back through the history of steel making, they were the last one to come to the table. And instead frankly, we're oftentimes looking for government to bail them out instead of innovating. And, and now they just got acquired by a giant Japanese corporation. So, you know, if you're looking for, my advice to, you know, these, these manufacturing clients is, if you're looking to to compete with the rest of the world. The rest of the world is looking at these same technologies. If you're looking to, to outcompete them. Then we, we've gotta be the country that's first to innovate on these things and first to try these things. There's way to de-risk this stuff. You don't have to bet the farm, on AI. There's, there's lots of ways to de-risk these types of projects, but to not, to not go after them at all, to not even, you know, dip your toe in and try some of these things and see what works is, is probably not the best approach.
Tom Raftery:Sure. Sure, sure. And looking ahead, what emerging technologies or strategies do you think would game changers in sustainable manufacturing?
Bryan DeBois:Yeah, I mean, I think this, this adoption of, you know, obviously I'm biased because I'm so focused in this right now, but I think this adoption of autonomous AI really saw it as a, I really do see it as an inflection point. I think AI in general, you know, one of the things that gets thrown a lot around a lot is that we're in the fourth industrial revolution, right? And so you can look back at all the different you know, the first through third revolutions, and you can see now, you can see, well, okay that, that, that revolution was about this, it was about, you know, moving from pneumatics to, you know, transistors and things. And you, it's hard when you're in the middle of a revolution to know. But, I really feel strongly that when we get to the end of the fourth industrial revolution, we'll look back and say, oh, that was to get to AI. When I started my career, we were still talking to customers about recording all the data coming from the plant floor and making sure that you're not throwing away all that valuable data. Now, in retrospect, I'm real glad we did that because AI's real hungry for data, right? So, so it does feel like we were building towards this AI future. So I see AI as, as the path forward. But then I think this Autonomous AI is a serious inflection point when you talk about being able to to perform as well as humans and in some cases outperform humans and find strategies that, that human operators and human supervisors wouldn't even see. I think that that's a big deal. As far as how that applies to sustainability. I think we're, you know, we're one of the, the handful of countries that still has a big manufacturing footprint, but also is, is looking probably the closest at sustainability. There's a lot of developing countries that have, you know, big manufacturing output but they're, you know, in a lot of ways, they're just trying to make stuff as fast as they can. And, you know, they're not even necessarily looking at the human costs. They're certainly not looking at ecological costs. So if we can combine our typical, you know, American innovation with AI, as well as our focus on sustainability, I really think that we've got everything we need to, to move us forward into a sustainable future.
Tom Raftery:Nice. Nice. We're coming towards the end of the podcast now. Bryan, is there any question that I haven't asked that you wish I had or any aspect of this we haven't touched on that you think it's important for people to think about?
Bryan DeBois:Yeah, I mean, I think my general advice to a company that maybe is thinking about this is, is looking at maybe, you know, dipping their toe into it, is to get a system integrator involved. I know that sounds self-serving. Even if it's not us, it's that important. Because when you just trust what the, AI vendors are telling you, you know, they, all they have is a hammer, so everything's gotta look like a nail to them. Whereas an independent system integrator can actually look at all the different platforms that are available. Can tailor a solution to what you actually need. And like I said, we've got a lot of, capabilities to de-risk these projects along the way so that you're not spending a ton of money, millions of dollars like some of the big, big IT consultancies will ask you to do, and then not really have anything to show for it at the end. So get an SI independent SI involved so hopefully someone who's very versed in the plant floor and that world of operational technology. It's just that important. and then just, just make the move, you know, do a small project. Do a pilot, things like that. But, start getting involved in that. Some of the most forward thinking companies that, that we're working with have titles like Director of Digital Transformation, VP of Industry 4.0. That to me. When I walk in there and I'm sitting down across the table from someone with a title like that, to me, that's the type of company that, you know that it feels like a forward thinking company. It feels like a company that's gonna last for a long time and you know, maybe not get bought out by a giant Japanese, you know, mega corporation.
Tom Raftery:Fair enough. Good, good, good. Bryan, if people would like to know more about yourself or any of the things we discussed in the podcast today, where would you have me direct them?
Bryan DeBois:you Yeah, so they can always find me on LinkedIn, so Bryan, B-R-Y-A-N-D-E-B-O-I-S, on LinkedIn. Or check us out at Rovisys.com/ AI. That's the AI section of our, of our website, and I always am happy to talk to anyone.
Tom Raftery:Fantastic. Bryan, that's been really interesting. Thanks a million for coming on the podcast today.
Bryan DeBois:Thanks Tom
Tom Raftery:Okay, thank you all for tuning in to this episode of the Sustainable Supply Chain Podcast with me, Tom Raftery. Each week, thousands of supply chain professionals listen to this show. If you or your organization want to connect with this dedicated audience, consider becoming a sponsor. You can opt for exclusive episode branding where you choose the guests or a personalized 30 second ad roll. It's a unique opportunity to reach industry experts and influencers. For more details, hit me up on Twitter or LinkedIn, or drop me an email to tomraftery at outlook. com. Together, let's shape the future of sustainable supply chains. Thanks. Catch you all next time.